In the swiftly advancing domain of artificial intelligence, the role of CLIP (Contrastive Language-Image Pre-training) models in computer vision has been both significant and revolutionary. Following our in-depth discussion on the architecture of CLIP models in the previous article, we now turn our attention to how one can fine-tune such sophisticated models.
Before diving in, if you need help, guidance, or want to ask questions, join our Community and a member of the Marqo team will be there to help.
1. What is CLIP?
Before diving into the specifics of training and fine-tuning, let’s revisit the key concepts behind CLIP models. Developed by OpenAI, CLIP represents a notable breakthrough in combining computer vision and natural language processing. It utilizes a large dataset of images paired with textual descriptions to train a model capable of understanding and correlating visual and textual information.
The fundamental innovation of CLIP lies in its ability to process both images and text into a shared embedding space. This is achieved through two main components: an image encoder and a text encoder. The image encoder converts images into embeddings, while the text encoder performs the same function for text. These embeddings are then aligned using contrastive learning, which brings the embeddings of matching image-text pairs closer together and pushes apart those of non-matching pairs.
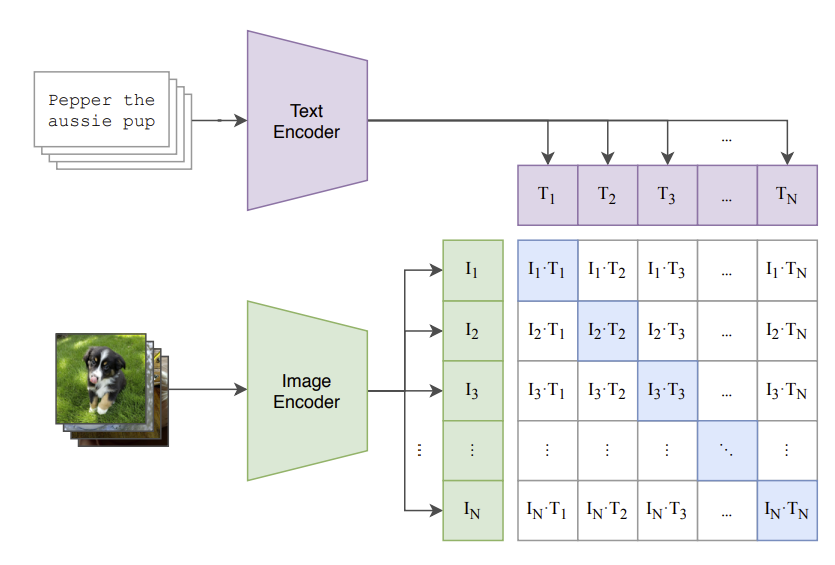
CLIP’s capacity to learn from extensive image-text pairs empowers it to perform a variety of tasks without needing task-specific fine-tuning. This versatility, coupled with its strong performance in zero-shot learning scenarios, makes CLIP an attractive choice for a myriad of applications, including image classification and object detection.
Let’s take a look at how we can fine-tune our own CLIP model for image classification!
2. Fine-Tuning CLIP Models
For this article, we will be using Google Colab (it’s free!). If you are new to Google Colab, you can follow this guide on getting set up - it’s super easy! For this module, you can find the notebook on Google Colab here or on GitHub here. As always, if you face any issues, join our Slack Community and a member of our team will help!
For this article, you will want to use the GPU features on Google Colab. We’d recommend changing your runtime on Google Colab to T4 GPU. This article explains how to do this.
Install and Import Relevant Libraries
We first install relevant modules:
Now we've installed the libraries needed to fine-tune, we must obtain a dataset to perform this fine-tuning.
Load a Dataset
This outputs:
Thus, the features of the dataset are as follows:
Cool, let’s look at the image!

As expected, it's an item of men's topwear.
We can see that the data itself is comprised of a train dataset, so we will define our dataset as this.
Awesome, so now we've seen what our dataset looks like, it's time to load our CLIP model and perform preprocessing.
Load CLIP Model and Preprocessing
Let's take a look at how well our base CLIP model performs image classification on this dataset.
This code uses the CLIP model to classify three example images from our dataset by comparing their visual features with textual descriptions of subcategories. It processes and normalizes the features of the images and subcategory texts, calculates their similarity, and predicts the subcategory for each image. Finally, it visualizes the images alongside their predicted and actual subcategories in a plot.
This outputs the following:

As we can see for the three images, our base CLIP model does not perform very well. It only identifies one of the three images correctly.
Let's set up the process for fine-tuning our CLIP model to improve these predictions.
Processing the Dataset
First, we must split our dataset into training and validation sets. This step is crucial because it allows us to evaluate the performance of our machine learning model on unseen data, ensuring that the model generalizes well to new, real-world data rather than just the data it was trained on.
We take 80% of the original dataset to train our model and the remaining 20% as the validation data.
Next, we create a custom dataset class:
Let's break this down:
Next, we create DataLoaders:
Here,
Next, we modify the model for fine-tuning:
Here,
Finally, we instantiate the fine-tuning model:
Here,
Amazing! We've set up everything we need to perform fine-tuning! Let's now define our loss function and optimizer.
Define Loss Function and Optimizer
We define as follows:
Here,
Great, now we set up the fine-tuning!
Fine-Tuning CLIP Model
We are now in a position to perform our fine-tuning. Let's break down the code in the training loop below.
Training:
Validation:
Here’s the full code:
Amazing! Each epoch takes around 3 minutes to run. Since we have 5 epochs, this code takes roughly 15 minutes so go grab yourself a cup of tea ☕️ and come back to see the magic of fine-tuning!
Here's a screenshot of the results we get once fine-tuning is complete. Note, you may get different results when running the code yourself.
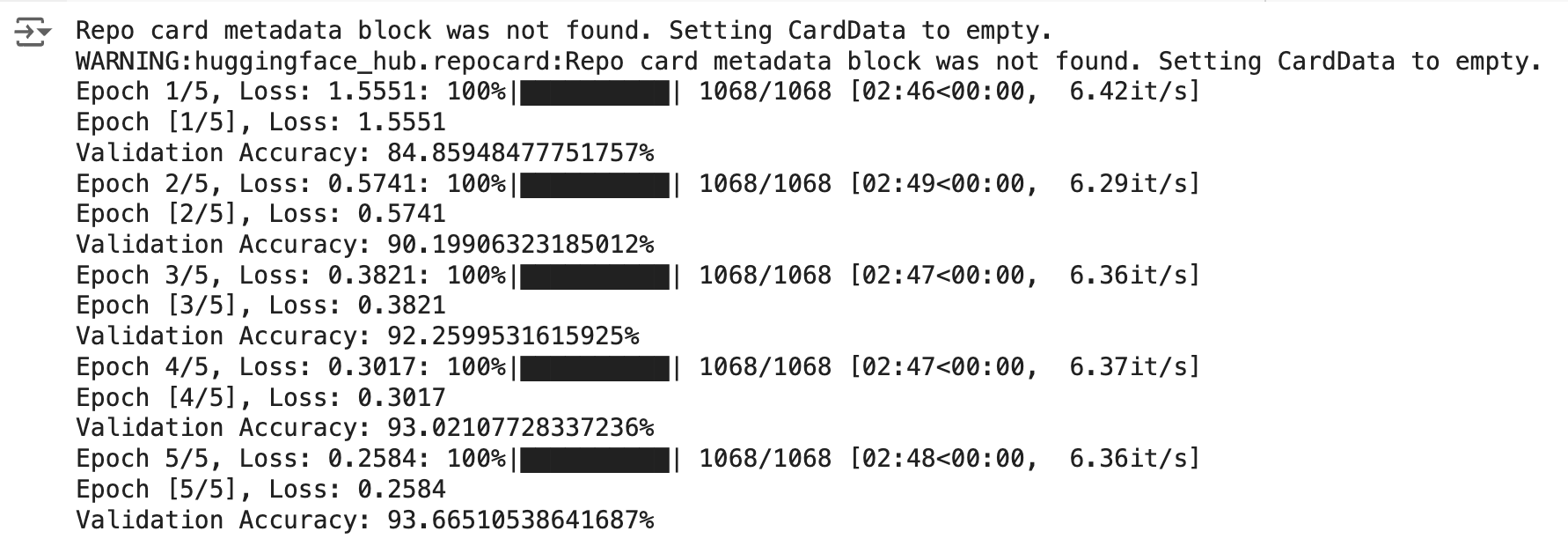
As you can see, the fine-tuning process is successful, with the model showing significant improvements in both training loss and validation accuracy across the epochs. The final validation accuracy of 93.67% is a strong result, indicating that the model has effectively learned from the training data and is performing well on validation data. The gradual decrease in training loss and steady increase in validation accuracy reflect a well-conducted training process with no signs of overfitting or underfitting.
Amazing! Let's now take a look at how our new model performs on the same images we tested earlier.
This returns the following:
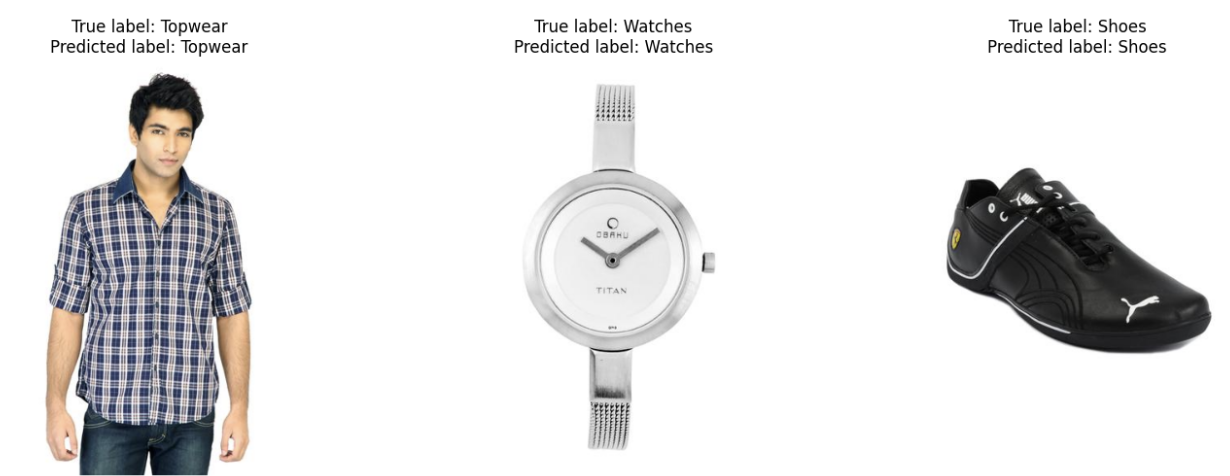
Super cool! Our newly fine-tuned CLIP model successfully predicts the labels for the three images!
Why don't you test out different images and settings to see if you can get even better results!
3. Conclusion
In this article, we successfully fine-tuned a CLIP model for image classification, demonstrating significant performance improvements. Starting with a pre-trained CLIP model, we utilized a fashion dataset and processed it to train the model effectively. Through careful dataset preparation, model modification, and training, we achieved high validation accuracy and improved predictions. This process highlights the power and versatility of CLIP models in adapting to specific tasks.
4. References
[1] A. Radford, et al. Learning Transferable Visual Models From Natural Language Supervision (2021)